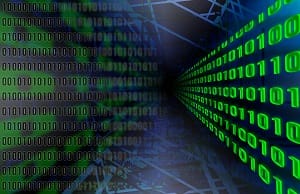
How Bad Data Hurts Quality Improvement in Healthcare
The U.S. Department of Health and Human Services defines Quality Improvement (QI) as systematic and continuous actions that lead to measurable improvement in health care services and the health status of targeted patient groups.
While procedures and systems are necessary to ensure continuity, equally important is the measurement of actions and patient health status, which require data not only to establish a baseline but also to continuously track, monitor and measure progress toward QI goals.
So, it stands to reason that data are vital to the success of QI initiatives. Data must not only be captured to track when and what actions were taken but also the result of the actions and the patient’s response. Without data, one could reasonably argue, QI programs cannot exist.
What is bad data, how does it happen and what’s the impact? [Bad Data in Healthcare]
Mostly, we think of bad data as data that contains errors. While erroneous data is certainly bad, it’s not the only kind of bad data. Data can be perfectly accurate but it can still be bad if it’s stored in a way that makes it difficult to access, requires further manipulation to be understood or if it’s accurate but incomplete.
Inaccurate data can result from:
- Typos
- Transcription errors
- Measurement errors
Data errors can lead to bad decisions, which adversely affects care quality. Also, if errors frequently occur, then trust of the data erodes. As clinicians’ trust of the official data falters, the more likely they are to create “shadow charts,” which in turn leads to other data problems – privacy concerns perhaps chief among them. In terms of QI, it’s clear that unofficial records can have a negative effect upon quality.
A good example of data that’s difficult to access are data stored in e-faxes, scanned documents, paper medical records or electronic data that’s not integrated. While these data may be accurate, if it’s difficult to access it’s at greater risk of being ignored. Clearly ignorance of data that may be helpful can lead to incorrect conclusions.
Data that’s accessible but requires altering it to understand or make use of it is also bad data. This can occur, for example, when data from a test result is rekeyed from a fax. It’s keyed into the proper EMR field but the unit measure or test name isn’t standardized. This can lead to interpretation errors when converting measures on-the-fly or even confusing one test for another. Again, quality suffers and efforts to improve are hindered.
Lastly, incomplete data is also bad. Using the same example of a fax lab result, some test components of a lab result may be rekeyed while others may be ignored. While the other components may not have been important at the time the data was keyed, it’s possible this data may be helpful in the future care of that specific patient or to understanding the incidence of a test measure across a patient population. In either case, quality suffers.
If you’re concerned about the accessibility, accuracy and/or completeness of clinical data and you’re concerned it may be hindering your QI initiatives, let us show you how our intelligent clinical data extraction solution can help you improve data integrity, accessibility and completeness.