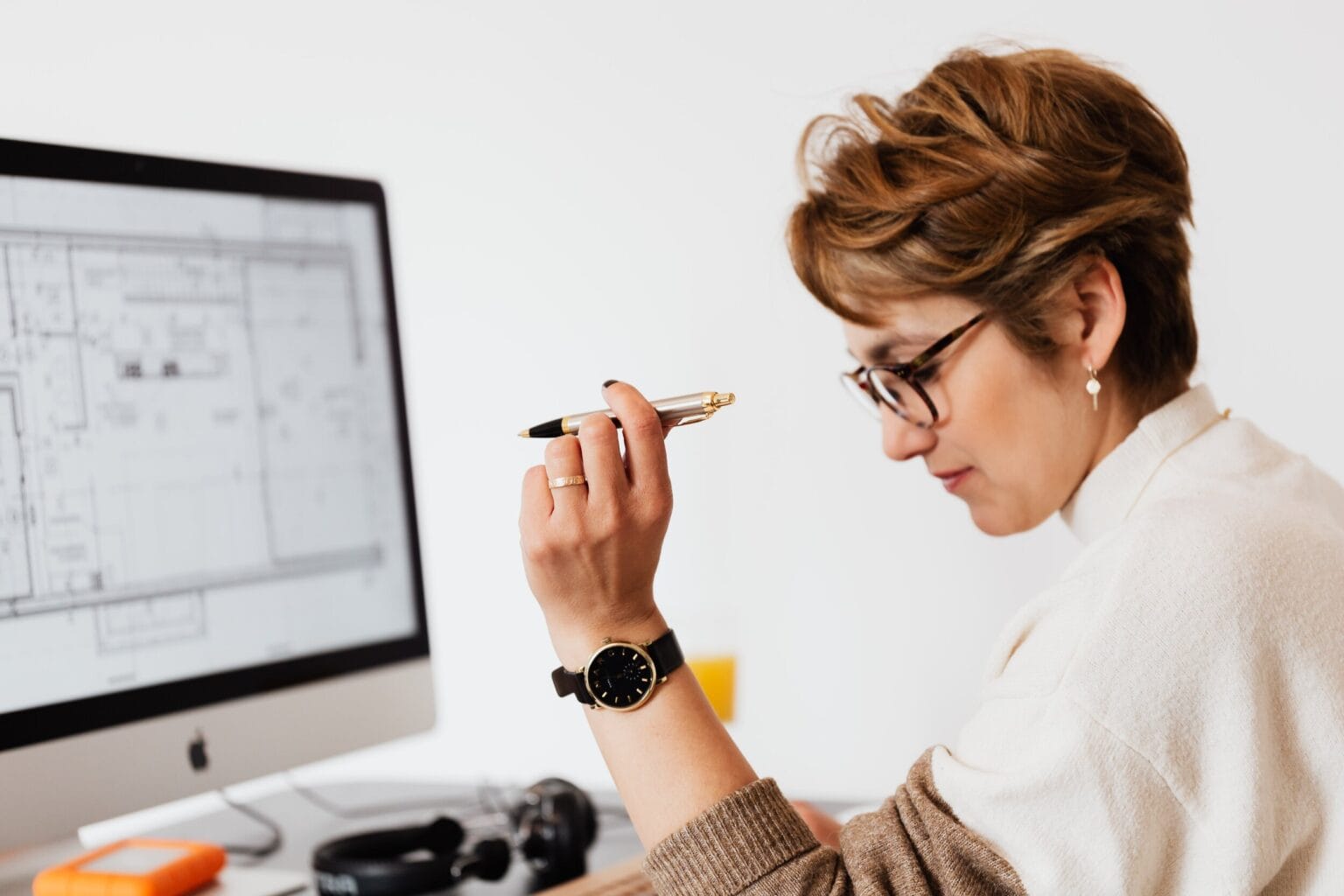
Decision Making in Healthcare
Healthcare decision making is an exercise in probabilities. Much effort has been expended to improve diagnosis and treatment decisions by making more and more data and data analytics available to the clinical staff. Decision making is probabilistic because it is impossible to tame all the variables affecting a patients physical and mental wellbeing. Doctors don’t know everything, but through experience, and I imagine through school training, clinicians deal with the uncertainty and the lack of definitive data to make decisions based on what they believe will produce the best outcome for their patients. It needs to be mentioned that this blog discussion focuses on hard data and ignores “soft” input from the patient. What the patient wants/needs can be ignored here, but clinicians do not ignore their patients in the real world.
There are concrete ways to help decision makers deal with the unknown. The analysis of large patient data sets where the details of a current patient can be compared to information tediously gathered from records on tens of thousands of previous patients. Amazon Healthlake contains information on 40,000 patients that spent time in critical care units. This reservoir of information contains demographics, vital signs, lab test results, medication, imaging reports, and provider notes and is being used to make predictions about the progression of disease, clinical trial efficacy and accuracy of insurance premiums. If one can predict the progression of disease and the outcomes of different treatment options, patients will benefit. So will insurance companies, pharmaceutical companies, payors, providers, and generally, all of society.
Gathering this data and making sure it is accurate is extremely difficult. Getting access to the data is difficult enough because the information is protected. Additionally, healthcare organizations haven’t fully figured out how to cost effectively gather and deidentify vast numbers of patient records. If money wasn’t a constraining factor, healthcare could hire all of the help, and buy all of the software, consulting, and hardware needed to build huge data sets. The reality is that healthcare organizations have to prioritize their spending and coming off a year’s long pandemic, management is being very selective in picking big projects. That means improvements are likely to be incremental and obtained by using or redeploying existing resources. Cost saving Initiatives or other patient care improvements are priorities and often, the projects that are lucky enough to be selected have to pay for themselves very quickly, if not in the immediate fiscal period.
Step one – gather the data and get it into the electronic record if it is not already in the electronic record. There are a plethora of data repositories in healthcare and bringing the data together is no simple task. A surprisingly large amount of “outside” critical data is coming to healthcare organizations in the form of faxes, paper, and scanned images which is not easily added to the electronic record. There are hundreds of different document types to process, some of which are extremely rich in patient information (e.g. laboratory test results and referral patient records) and must be entered.
It is expensive to process these incoming documents manually. Over time, strategies have been implemented to reduce these costs, including the implementation of interfaces (high upfront cost) and outsourcing of the document handling workflow (high long-term cost plus a lack of control of the data). Another alternative is to purchase automated document and data handling software that significantly reduces manual efforts, reduces data errors found in the EMR, and speeds the turnaround time so that the data is available to physicians more quickly. Extract has hundreds of successful implementations of workflow automation.
Extract uses a long list of tools and technology to improve workflows. Of all the various benefits of automation, like reduced costs and faster turnaround times, the most critical aspect of these workflows is accuracy. Without accuracy, an automated workflow is dangerous. Extract is relentless when it comes to calculating and tracking accuracy of both the software and of the post process accuracy as the data is delivered to the EMR.
It is important to reduce the clinician’s reliance on probabilities and that can only be done by providing accessible, affordable, and accurate data.